Data Cleaning Best Practices For a Better Database
Last updated: May 15, 2024
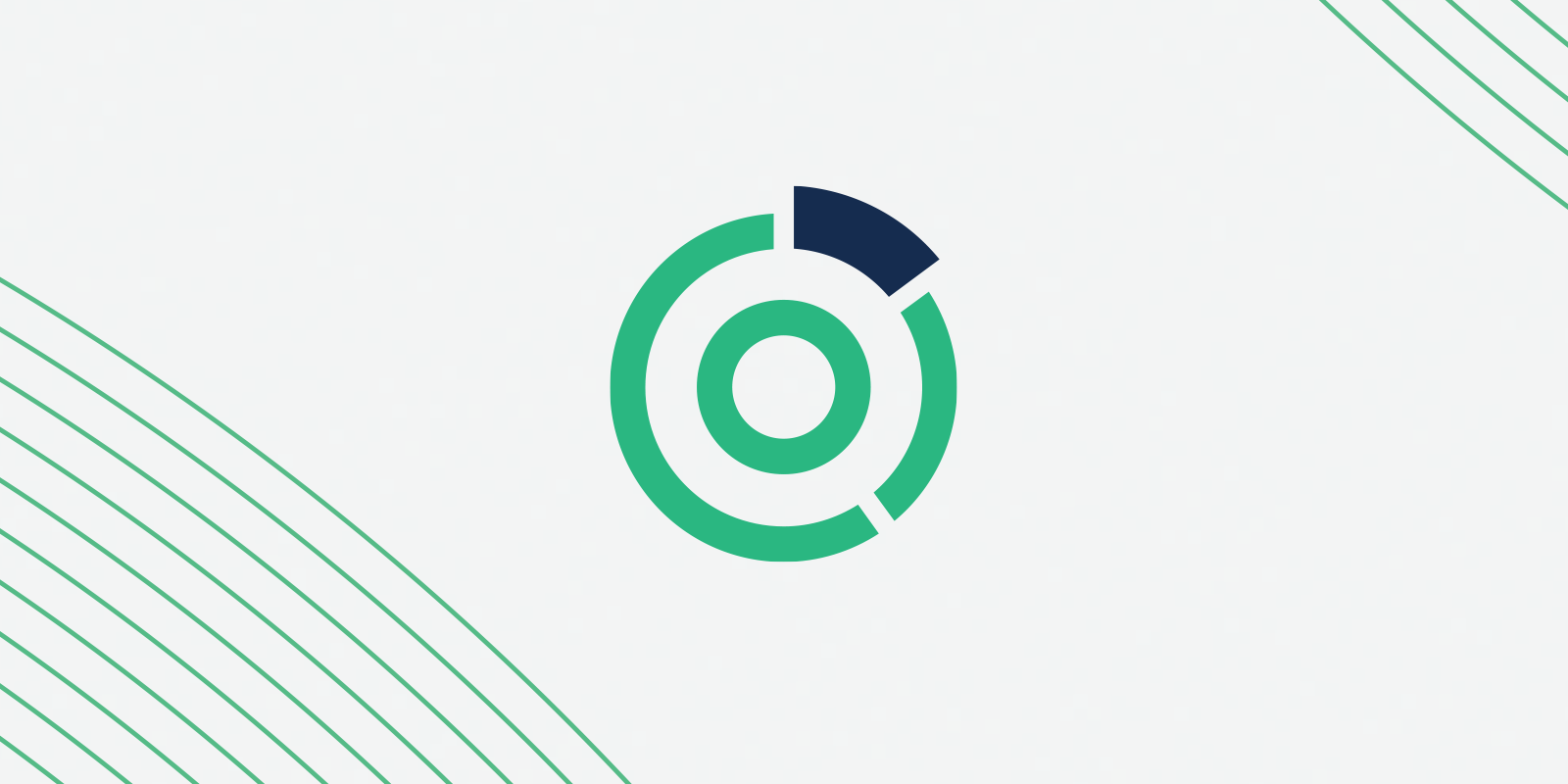
Data cleaning is essential for ensuring that your customer data is actionable and easy to use.
Unfortunately, many marketers fall short on that front: 45% of marketers don’t validate their data for quality and accuracy, and 62% use incomplete or invalid prospect data, according to research from Mercury, a creative digital marketing agency.
That’s bad news, because inaccurate prospect data can undermine your marketing campaigns before they even begin.
Streamline your tech stack: Boost efficiency, unify data & retain talent! Get expert tips now:
There’s the obvious consequence – not being able to reach your prospects at right email address. But if you never check your contact lists for accuracy, you might also assume that your campaign’s failure is due to poor strategy, rather than an outdated contact list. This can lead you to make unnecessary changes to your strategy and set you back even further.
And without an accurate view of each customer, you can’t customize your content and offerings to their needs.
So how can you make sure your data always reflects what’s actually going on with your audience? Here, we’ll explain what it means to have “clean” data, then present data hygiene best practices and cleaning data sets.
What does it mean to have “clean” data?
Data cleaning is the process of fixing or removing incorrect, corrupted, incorrectly formatted, duplicate, or incomplete data within a dataset. So data is “clean” when:
- You can establish a single view of each customer (i.e., you don’t have any duplicates)
- Each customer’s record is accurate and up to date
- Each data label is standard across every software you’re using (i.e., you use DD/MM/YYYY across all platforms rather than mixing it up between tools)
- It’s error-free, without any typos, structuring or formatting issues
- If you’re using third-party integrations to send data between systems, imported data is being stored under the right label (i.e., your data mapping is accurate)
Data cleansing and standardization best practices
One of the biggest barriers to data hygiene is the sheer scope of data to manage. The average company takes in customer data from a rapidly increasing amount of marketing channels, like email, social media, website forms and tracking, third-party cookies, etc.
And when your data consumers/stakeholders are siloed on different teams, it’s possible that nobody in your company knows exactly where all of the data comes from, much less how to wrangle it and make it actionable.
1. Audit your data
Performing a regular data audit can help you keep track of your data – and diagnose potential quality issues. Locate all of your existing data, list your input and output data sources, and identify any recurring issues that might impact your data quality.
2. Standardize data at the point of entry
Data is only actionable when every stakeholder knows what exactly each datapoint is measuring. This is only possible when every data point is standardized from the first point of entry.
Establish a set of “brand guidelines” for data labeling so every data point is uniform and can be easily used across teams. This will reduce the need for data cleaning after it’s collected – and reduce the likelihood of quality issues.
For example, you might use a prospect’s local time zone to measure when they took a particular action, rather than GMT, etc. Or you might agree on a certain set of criteria to move each candidate’s record from your CRM to your customer data platform, etc.
You should also create a standard operating procedure for data entry, especially if some points are still entered manually. Also implement regular process checks into the process so that errors can be resolved before they impact campaign success or the health of your database.
Obviously, you can’t manually check whether each of your customers have changed job titles or email addresses in the next 6 months. But you can periodically review your database to confirm that your customer records are legitimate and free of irregularities.
Customer data platforms like Omeda can perform many of these processes automatically. To flag fake accounts, Omeda checks the incoming name fields of each profile and rejects any name that has no vowels, 6+ consecutive consonants, repeating letters or is on a list of specific names that have been marked as junk.
Omeda has many built-in data cleaning processes that make this process automatic: For instance, we have built-in procedures to remove junk characters (* or !, etc.) from customer profiles and match incoming information to established customer profiles.
Omeda and other customer data platforms also require that specific data fields be mapped (like email, one part of a postal address, etc.,), all of which keeps customer data as accurate and relevant as possible.
3. Remove duplicate records
When customer data is spread across multiple software, it’s more likely that you’ll accidentally create more than one customer record for the same person. This could happen if someone books a demo, which is tracked by your CRM, but also regularly browses your website (which is recorded by your CDP or another web tracking tool).
Duplicate records don’t just complicate your reporting and making your campaigns. But they also prevent you from getting a single view of your customer and reaching them effectively. Given the marketing and reputational cost of dupes, you should look to merge duped records whenever possible.
But what if you don’t want to risk losing data from one of the records? Or you can’t be sure that two similar records belong to the same person?
Customer data platforms can help you identify and merge identical customer profiles without losing any profile data, For instance, Omeda’s identity resolution solution uses a combination of exact and fuzzy matching to develop a confidence score for determining unique and common records. If the records are determined to belong to the same person, the data from both profiles is merged into one, with any outdated information archived in our database for record-keeping.
4. Simplify your tech stack
Many data quality issues stem from overcomplicated tech stacks. Data stored in one place never makes it to the other, or it arrives with inconsistent naming conventions or incomplete records. This makes it a lot more difficult to use that data for its intended purpose, whether that’s creating segments, personalizing messaging, etc.
Multiply that over thousands of customers and it has huge revenue and opportunity costs for your business
Solve that problem by consolidating your tech stack. This makes it easier to manage, activate and benefit from the customer data you work so hard to collect. For instance, Omeda’s form builder connects to our customer data platform. This way, every form response is automatically recorded in our customer database, instead of needing to be cleaned, manipulated and sent across teams.
Instead, sales and marketing can find it right away, capitalizing on audience interest more quickly, and ultimately get more conversions..
So as you evaluate your data strategy, look for opportunities to streamline. If you’re doing your email marketing in one platform and marketing automation in another, consider managing both processes in one audience management platform.
Subscribe to our newsletter
Sign up to get our latest articles sent directly to your inbox.
What you should do now
- Schedule a Demo to see how Omeda can help your team.
- Read more Marketing Technology articles in our blog.
- If you know someone who’d enjoy this article, share it with them via Facebook, Twitter, LinkedIn, or email.